The buzz around artificial intelligence has reached fever pitch. In an Oliver Wyman-New York Stock Exchange Survey of executives from NYSE-listed companies, 96% said they viewed AI as an opportunity for their business, not a risk. Drilling down to respondents from health and life sciences companies, 100% ranked AI as an opportunity.
Healthcare respondents were especially optimistic about AI’s potential to improve workforce productivity (88%) and operational efficiency (75%). Most of those gains are likely to come through automation of burdensome manual tasks, but there are also upsides on the clinical front, ranging from bolstering diagnostic capabilities to delivering personalized treatment recommendations that can improve health outcomes and medication adherence, reduce unnecessary utilization, and help people live longer.
While AI offers significant potential, executives must navigate the technical, operational, and regulatory challenges of adoption. Proactive planning and strategic foresight are essential to mitigate risks and capitalize on AI’s transformative power.
The core principles of AI
Although variations of AI — machine learning, natural language processing, and now generative AI — have been used to varying degrees in healthcare for years, broad adoption remains low. An estimated 5% of healthcare and social assistance organizations leverage AI to produce goods and services, according to a recent analysis from the US Census Bureau. To be sure, other surveys suggest higher usage, but the fact remains that significant opportunities exist for broader and impactful use of AI in healthcare.
Exhibit 2: Understanding the types of AI

Source: Oliver Wyman
Transformative potential of AI in health system and payer operations
We’ve zeroed in on three dimensions where AI can be leveraged to transform payer and provider operations: process automation, personalized engagement, and decision support. Using AI in each of these areas will impact different aspect of care delivery, from follow-up care to actively monitoring a patient’s condition.
Exhibit 3: The potential for AI to transform operations
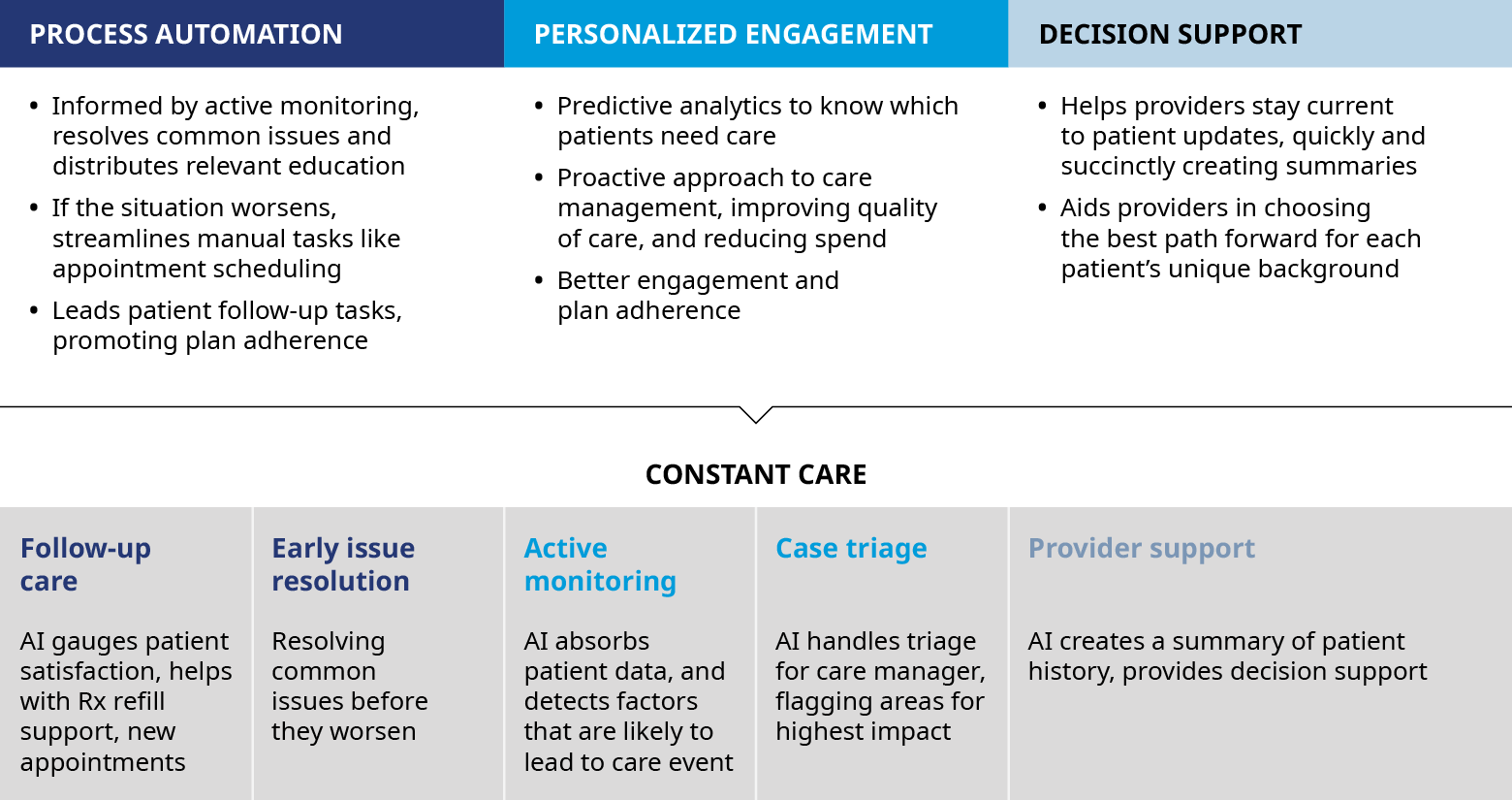
Source: Oliver Wyman
Process automation removes tedious, time intensive activities and eases staff burden, freeing up administrative or mid-level clinical staff to focus on direct patient care and interactions.
Let’s look some oft-criticized processes — prior authorization, claims processing and reporting:
Prior authorization and subsequent physician appeals currently involve numerous clinical support staff who must combine data from disparate sources into summaries for clinicians. Generative AI can look through patient charts and pull the most relevant data into a consolidated summary. This process automation may enable healthcare organizations to optimize their workforce, allowing payers and providers to allocate staff to more value-added roles. In addition, the use of AI removes the standard ramp-up of productivity for new hires, eliminating a large driver of turnover costs and reducing the chance of human error.
Exhibit 4: How AI can accelerate prior authorization
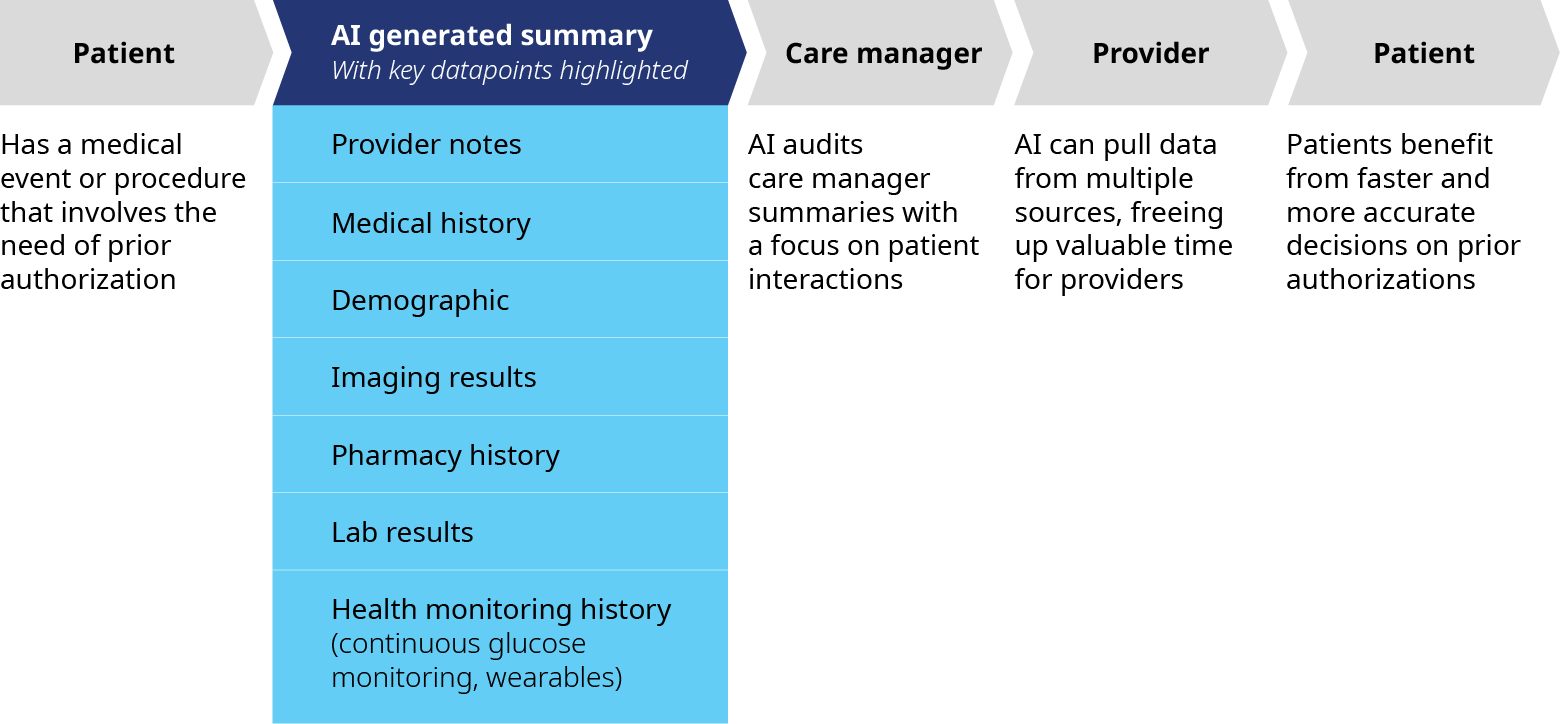
Source: Oliver Wyman
Claims processing and reporting also present compelling application for process automation. The claims ecosystem involves both data scrubbing to ensure accuracy and the movement of geographic, clinical, and plan-level data into a singular tube of information which is then used to determine what to pay towards a claim. Select outputs are then sense checked by an analyst before being passed further into the payer system. Machine learning can be used to create more sophisticated interfaces that pull this key data to the forefront for human reviewers. This, in tandem with decision validation, allows reviewers to be more efficient and accurate.
Another key application of AI centers on care management reports for patients with polychronic conditions and multiple episodes of care. These high-risk patients generate large amounts of data, spread across a range of provider notes: specialist, primary care physician, acute care, pharmacy, lab, imaging, and more. Care and case managers typically review these massive tranches of data and reports to glean critical inputs to inform care management. AI technology can rapidly review these datasets by searching for keywords and phrases to identify, isolate, and summarize the most critical insights and data across these various sources, reducing the admin burden of the human reviewer.
Improving outcomes through decision support
Machine vision, a critical component of AI, is set to revolutionize healthcare with its advanced capabilities. Aside from its current use in radiology, there are numerous other opportunities in healthcare where machine vision can play a role, enhancing both clinical and operational aspects for health systems and payers.
Radiologists are under immense pressure due to the increasing volume of medical imaging. The sheer number of scans can lead to fatigue, increasing the risk of errors and delays in diagnosis. In fact, nearly half of US radiologists said they were burned out, according a survey in the Journal of the American College of Radiology.
Machine vision can serve as an invaluable assistant, analyzing images with remarkable precision and speed. AI-powered imaging systems can identify anomalies and flag potential issues, allowing radiologists to focus on more complex cases. For image guided radiotherapy of head, neck, and prostate cancers, oncologist manually contour tumors from surrounding organs. Through the utilization of auto segmentation models, time spent on contouring can be reduced by up to 90%. This collaboration between AI and radiologists can enhance diagnostic accuracy, reduce workload, and accelerate treatment decisions, ultimately improving patient outcomes.
There are also opportunities to improve patient monitoring. Traditional methods rely heavily on periodic checks, which can miss subtle but critical changes in patient’s condition. This gap often leads to delayed interventions and subpar clinical outcomes. Machine vision can play a pivotal role by enabling real-time remote patient monitoring. These systems can detect changes in vital signs or movement patterns, alerting providers before conditions worsen, thereby improving patient outcomes and reducing readmissions.
Driving personalized care decisions
Nearly across the board, we’ve heard how copilot style tools can provide decision support and help physicians offer more precise care. These tools take advantage of predictive analytics to recommend the next action in a treatment plan. Providers can and often do consider patient background and information but are sometimes only educated on the best course of action for a typical patient, not always their patient.
For example, if a diabetic has not filled their prescription in the past four weeks, an AI tool can automatically flag this risk factor to a care manager, rather than the care manager having to sift through the data themselves. For a care manager with 100 patients, AI can both prioritize patients in a queue based on risk and recommend next steps to improve FTE efficiency and performance. Many physicians already utilize simple versions of this functionality through their provider organization’s electronic medical records system. Patient files, suggested treatment pathways, and potential medical interventions sit within the reach, but knowing how to access and interpret this data can place a burden on clinicians. AI functionality can present summaries and suggested treatment pathways that are tailored to a patient’s unique symptoms, coverage, and side effect tolerance. This reduced administrative burden frees the physician to devote more time to patient care.
Overcoming barriers to effective AI use in healthcare
While AI provides great promise in transforming the future of healthcare, complex legal and regulatory challenges, as well as technology issues, will need to be addressed. Use cases in clinical settings can expose both AI vendors and the clinicians who use them to regulatory or legal action. The Federal Trade Commission, for instance, warned that it would pursue cases against companies that loosen data policies to allow for greater mining of customer data. Cybersecurity is also a top priority in healthcare. The Department of Health and Human Services found that over 32 million patients were affected by cyber hacks in 2022. Organizations need to establish protocols to minimize the risk of a breach before downloading apps onto their networks and devices.
Moreover, many of these tools are in varied states of development. There are no clear champions of AI for healthcare, and many organizations must weigh the cost and return on investment of development. While high-quality and reputable vendors will likely emerge over time, homegrown solutions are commonplace—and expensive. Smaller payer and provider networks will struggle to keep up with large national organizations, and in turn, will be more hesitant to invest.
AI is not perfect. It may contain errors which can lead to confusion, wasteful spending, sub-optimal recommendations, or inaccurate reports. Clinical diagnostics are particularly vulnerable to quality issues; the Food and Drug Administration dedicates significant resources to investigate these programs. For example, in radiology, programs may miss abnormalities that trained physicians can still detect, while others present with a high degree of false positives. The former could lead to a life-threatening lack of treatment while the latter could place an unneeded burden on patients and physicians. Managing for inaccurate or biased results is essential.
By approaching investments in and adoption of AI with a focus on the highest impact use cases, executives can position their organizations at the forefront of technological innovation and improve overall healthcare delivery.
John Lester, Partner, Digital and Health and Life Sciences, contributed to this article.