Artificial intelligence (AI) promises to provide staggering gains in efficiency and output for organizations across industries that implement it properly. Based on analysis conducted by the Oliver Wyman Forum, AI will contribute to a 40% increase in labor productivity by 2035 in developed countries, stemming primarily from advancements in the end-to-end operations value chain. A key area of this innovation wave is the integration of AI into core operations planning functions, including long- and short-range production and maintenance planning, materials planning, and production control.
Traditionally, planning functions have heavily relied on the expertise and accumulated knowledge of human planners, who must navigate extensive volumes of operational data that require interpretation and analysis to create a plan, all while striving to strike a balance between costs and operational performance.
In today's highly complex and dynamic operational environments, this becomes an increasingly challenging task for humans alone. As a result, plans tend to be static and fall short of optimal, leading to errors and inefficiencies in downstream operations. For example, in the aviation industry, maintenance planners must sift through various systems and sources of information to build and refine the overnight aircraft maintenance. Misjudging maintenance durations or planning work at an inadequately resourced location can quickly result in flight delays or cancellations.
While the availability of advanced planning solutions has helped mitigate some of these challenges, human involvement and errors remain prevalent throughout the planning process. AI — which excels in processing vast datasets, operating in real-time, and handling tasks that require forecasting and combinational reasoning — is particularly well-suited to address that problem. Organizations that harness these capabilities can elevate their planning processes, resulting in heightened efficiency, minimized errors, and improved operational performance. Our experience shows that the application of AI in planning can lead to a 40% reduction in the planning effort while halving planning errors.
Embedding AI in the planning process for enterprise resource planning systems
In the traditional planning process, the planner researches the current situation, troubleshoots, and formulates potential solutions. Finally, the planner updates the plan or carries out the final decision by executing the necessary transactions in the enterprise resource planning (ERP) system. For instance, in preparation for the peak shipping season, a shipping company's planners work on optimizing container utilization. They assess the current situation by reviewing bookings, container availability, and external factors, then look for inefficiencies such as overbooked routes and underutilized containers. Solutions like consolidating shipments and adjusting schedules are developed. The planners then implement the chosen strategies through the company's ERP system, which involves updating shipping routes and schedules, communicating changes to customers, and coordinating with ports for efficient transitions.
In most organizations, these steps are plagued with non-value-added activities including researching multiple data sources to understand scope, resources, capacity, and other operational parameters. Many of these tasks can be automated using software applications commonly known as "AI agents" that can mimic human behavior. Such agents can proactively address exceptions and continuously improve the planning process through self-learning and feedback from the supervising planner. Exhibit 1 illustrates how incorporating AI agents into the planning process can generate plans faster , with greater accuracy and fidelity, allowing the planner to focus on exceptions and active disruptions.
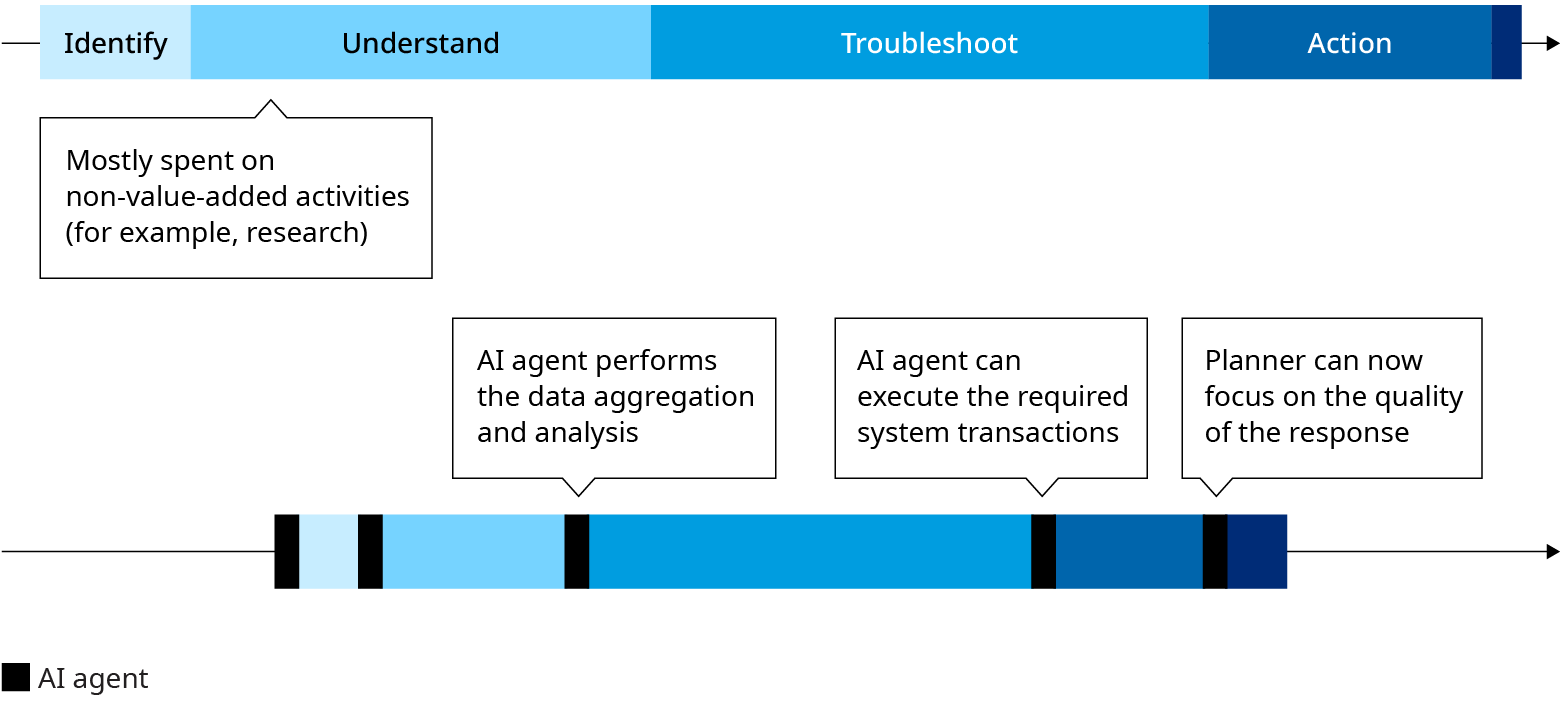
Five stages of planning maturity towards full automation
It comes as no surprise that planning is moving toward full automation. However, due to the limitations of existing infrastructure, this transition is more of a marathon than a sprint. Many organizations are relying on planning solution providers that have promised to incorporate AI into their offerings in the coming years. Others are developing their own planning agents to emulate the role of a planner. Either way, the journey to fully automated planning involves a series of progressive steps that not only enhance productivity over time but also bolster trust in the technology at its core. This maturity model can be conceptualized as consisting of five distinct stages.

Manual task execution in planning
Planners perform most tasks, from the gathering of decision-critical information to the execution of transactions. For example, reviewing current inventory counts and comparing them to historical demand.
Informed decision-making in planning
Planners are provided with improved access to data through tailored dashboards and reports, to support sound operational decisions.
Assisted operations in planning
Planners use tools that perform data interpretation on their behalf, and offer direct recommendations on optimal actions — for instance, how best to remedy an inventory shortfall.
Supervised processes in planning
Solutions begin to carry out transactions within the system under the planner’s oversight and approval.
Automated systems in planning
The solutions take the reins on the majority of transactions autonomously, only deferring to human judgment in exceptional cases.
Practical AI solutions for planning
Generative AI and machine learning models have demonstrated strong capabilities in summarization and extraction, searching with scale, and recommendation development, making them perfect companions on the path to full automation. Companies are starting to deploy them in a variety of creative ways:
Retrieval Augmented Generation (RAG) techniques
RAG techniques are commonly used for assistant chatbots, as a supercharged internal search engine that rapidly query vast amounts of structured and unstructured data to provide precise answers to user questions. For instance, in inventory planning, a chatbot assistant is connected to the company’s operational data stores and internal policies and manuals. The bot acts as a single point of contact for information and provides immediate assistance, responding to plain language questions such as “Do we have part number 01921 available in the New York area?” or “What parts are required to complete this maintenance task?”
Machine learning models
Machine learning models have demonstrated their ability to effectively identify patterns in extensive datasets, making them valuable tools in operational environments where they can be deployed and trained on historical decisions, actions, and events. These models are now commonly used in supply chain such as for inventory forecasting, predictive maintenance, and other pattern-driven applications. For example, in logistics, machine learning solutions can look at historical data on the dimension and weight of freight, traffic, fuel consumption, and weather to dynamically suggest optimal delivery routes.
Combining data analytics with generative AI
When data analytics are combined with generative AI, tools can be built to perform the necessary research and analysis to assess all possible options prior to making a planning decision, giving a human operator an opportunity to review the recommended course of action, before automatically executing the necessary steps. One such AI agent has been effectively used in commercial aviation to accelerate the “Aircraft on Ground” (AOG) process, where an aircraft is out of service due to a need for a spare part that isn’t available locally (Exhibit 3). The agent can automatically review inventory across the network to find the closest parts, check upcoming flights to see how soon the part can be transferred, search for potential alternates, and contact partner airlines to explore options for a part loan. The agent can then highlight the best option and execute the necessary transactions in the ERP system once approved by the human supervisor.

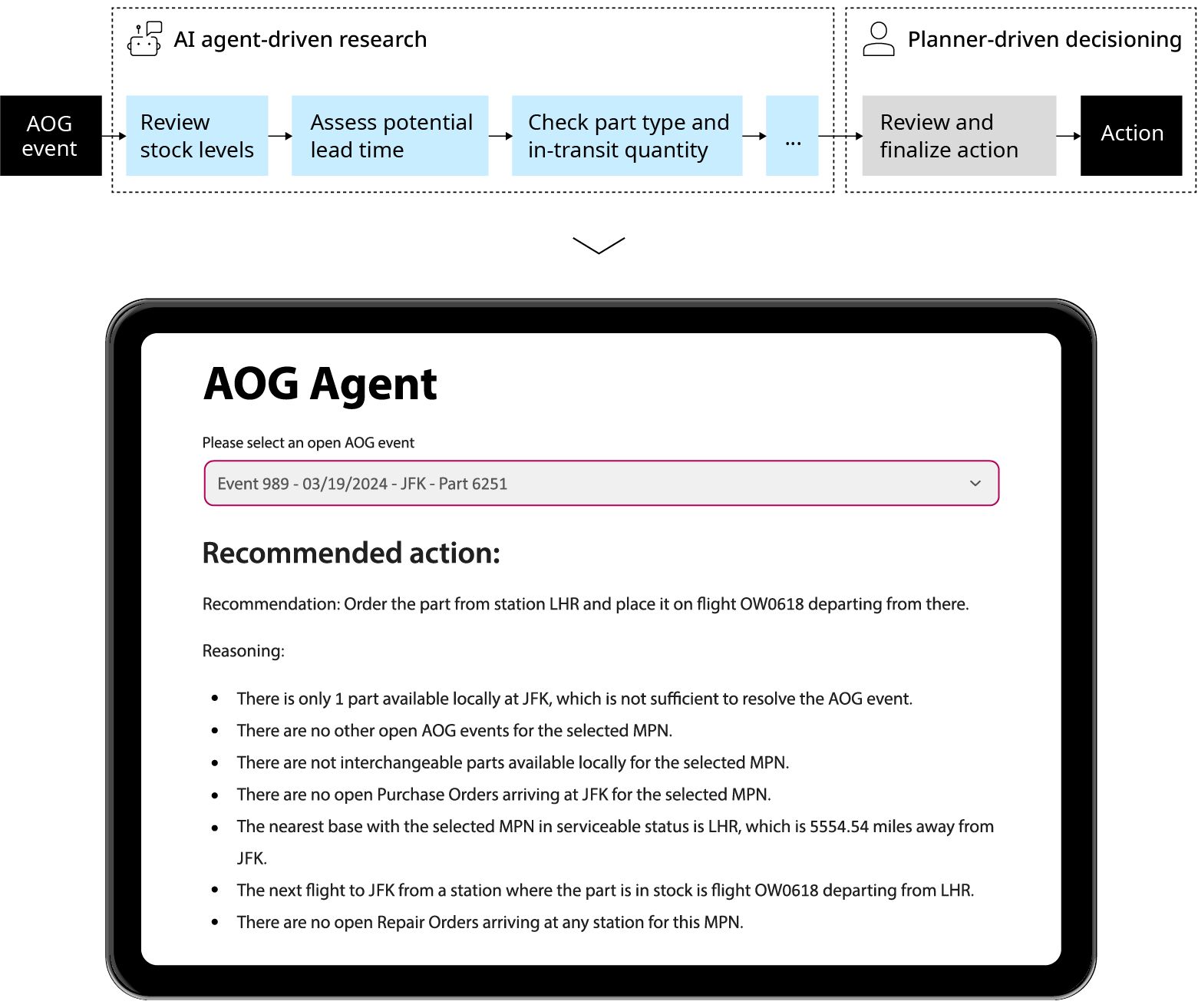
AI adoption in planning still faces challenges
When it comes to development of AI, like with most novel technologies, the learning curve is steep. Organizations are required to build their data science skillset around their planning functions, which includes modeling, training, fine-tuning, and productizing AI-based models. These tasks can be highly technical and may fall outside the core competencies of most organizations. Additionally, the development of AI models often requires multiple prototyping cycles, and the right infrastructure needs to be in place to support it. This includes considerations such as high-performance computing resources, scalable storage, and robust data management systems. Furthermore, the underlying business data needs to be clean and have sufficient historical information to effectively train the AI models. To fast-track the process, organizations may look to hire internal data scientist and development teams, or to bring in external partners to support with initial tools.
When it comes to the adoption of AI in planning functions, there is understandable skepticism within the operations community. Human judgment, creativity, and contextual understanding remain vital in many aspects of the planning process. However, there is a fundamental question regarding whether planning is solely an engineering function, where plans are simply the result of a calculated equation without any “art” involved. For now, to ensure long-term success, organizations must prioritize successful deployment and adoption by striking the right balance between AI and human involvement.